注:最近一段时间,AI作画的话题在整个人工智能圈子很是热门。当然,作画只是其中一方面,人工智能在生成内容这一块还有很多的应用场景,除了作画,还可以生成文字、生成音视频等。不久前,红杉美国特地将这个话题拎出来讨论了一番,非常有趣的是,这篇文章除了Sonya Huang和Pat Grady两位署名作者外,还有一个作者是GPT-3。本文前半部分是红杉的英文原文,后半部分为中文编译,希望对你有所启发。
本文来自:红杉,编译:深思圈,原文标题:Generative AI: A Creative New World,题图来自:《爱,死亡和机器人》
Humans are good at analyzing things. Machines are even better. Machines can analyze a set of data and find patterns in it for a multitude of use cases, whether it’s fraud or spam detection, forecasting the ETA of your delivery or predicting which TikTok video to show you next. They are getting smarter at these tasks. This is called “Analytical AI,” or traditional AI.
But humans are not only good at analyzing things—we are also good at creating. We write poetry, design products, make games and crank out code. Up until recently, machines had no chance of competing with humans at creative work—they were relegated to analysis and rote cognitive labor. But machines are just starting to get good at creating sensical and beautiful things. This new category is called “Generative AI,” meaning the machine is generating something new rather than analyzing something that already exists.
Generative AI is well on the way to becoming not just faster and cheaper, but better in some cases than what humans create by hand. Every industry that requires humans to create original work—from social media to gaming, advertising to architecture, coding to graphic design, product design to law, marketing to sales—is up for reinvention. Certain functions may be completely replaced by generative AI, while others are more likely to thrive from a tight iterative creative cycle between human and machine—but generative AI should unlock better, faster and cheaper creation across a wide range of end markets. The dream is that generative AI brings the marginal cost of creation and knowledge work down towards zero, generating vast labor productivity and economic value—and commensurate market cap.
The fields that generative AI addresses—knowledge work and creative work—comprise billions of workers. Generative AI can make these workers at least 10% more efficient and/or creative: they become not only faster and more efficient, but more capable than before. Therefore, Generative AI has the potential to generate trillions of dollars of economic value.
Why Now?
Generative AI has the same “why now” as AI more broadly: better models, more data, more compute. The category is changing faster than we can capture, but it’s worth recounting recent history in broad strokes to put the current moment in context.
Wave 1: Small models reign supreme (Pre-2015) 5+ years ago, small models are considered “state of the art” for understanding language. These small models excel at analytical tasks and become deployed for jobs from delivery time prediction to fraud classification. However, they are not expressive enough for general-purpose generative tasks. Generating human-level writing or code remains a pipe dream.
Wave 2: The race to scale (2015-Today)A landmark paper by Google Research (Attention is All You Need) describes a new neural network architecture for natural language understanding called transformers that can generate superior quality language models while being more parallelizable and requiring significantly less time to train. These models are few-shot learners and can be customized to specific domains relatively easily.
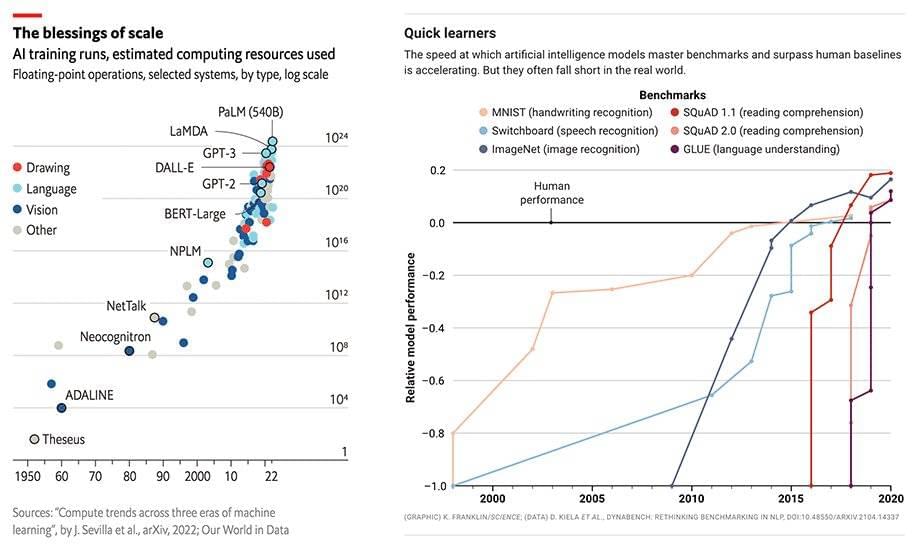
Sure enough, as the models get bigger and bigger, they begin to deliver human-level, and then superhuman results. Between 2015 and 2020, the compute used to train these models increases by 6 orders of magnitude and their results surpass human performance benchmarks in handwriting, speech and image recognition, reading comprehension and language understanding. OpenAI’s GPT-3 stands out: the model’s performance is a giant leap over GPT-2 and delivers tantalizing Twitter demos on tasks from code generation to snarky joke writing.
Despite all the fundamental research progress, these models are not widespread. They are large and difficult to run (requiring GPU orchestration), not broadly accessible (unavailable or closed beta only), and expensive to use as a cloud service. Despite these limitations, the earliest Generative AI applications begin to enter the fray.
Wave 3: Better, faster, cheaper (2022+) Compute gets cheaper. New techniques, like diffusion models, shrink down the costs required to train and run inference. The research community continues to develop better algorithms and larger models. Developer access expands from closed beta to open beta, or in some cases, open source.
For developers who had been starved of access to LLMs, the floodgates are now open for exploration and application development. Applications begin to bloom.
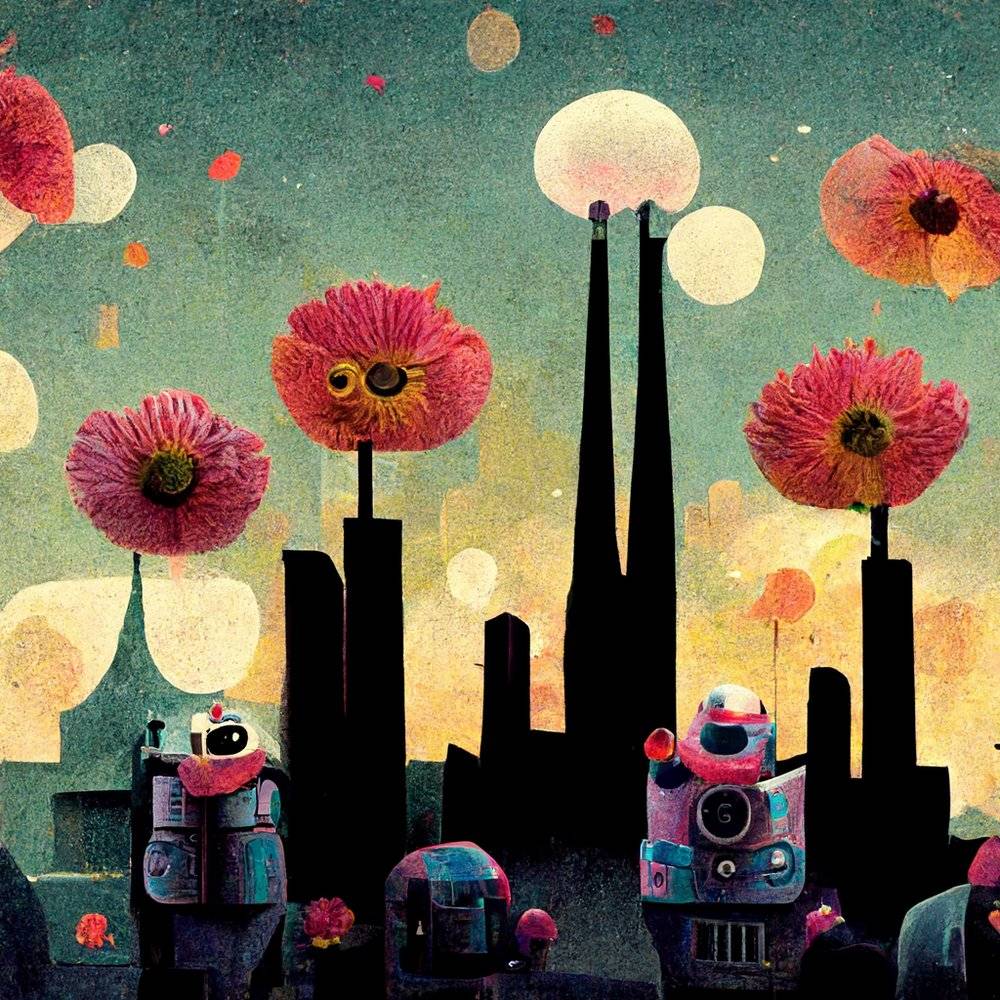
Wave 4: Killer apps emerge (Now) With the platform layer solidifying, models continuing to get better/faster/cheaper, and model access trending to free and open source, the application layer is ripe for an explosion of creativity.
Just as mobile unleashed new types of applications through new capabilities like GPS, cameras and on-the-go connectivity, we expect these large models to motivate a new wave of generative AI applications. And just as the inflection point of mobile created a market opening for a handful of killer apps a decade ago, we expect killer apps to emerge for Generative AI. The race is on.
Market Landscape
Below is a schematic that describes the platform layer that will power each category and the potential types of applications that will be built on top.
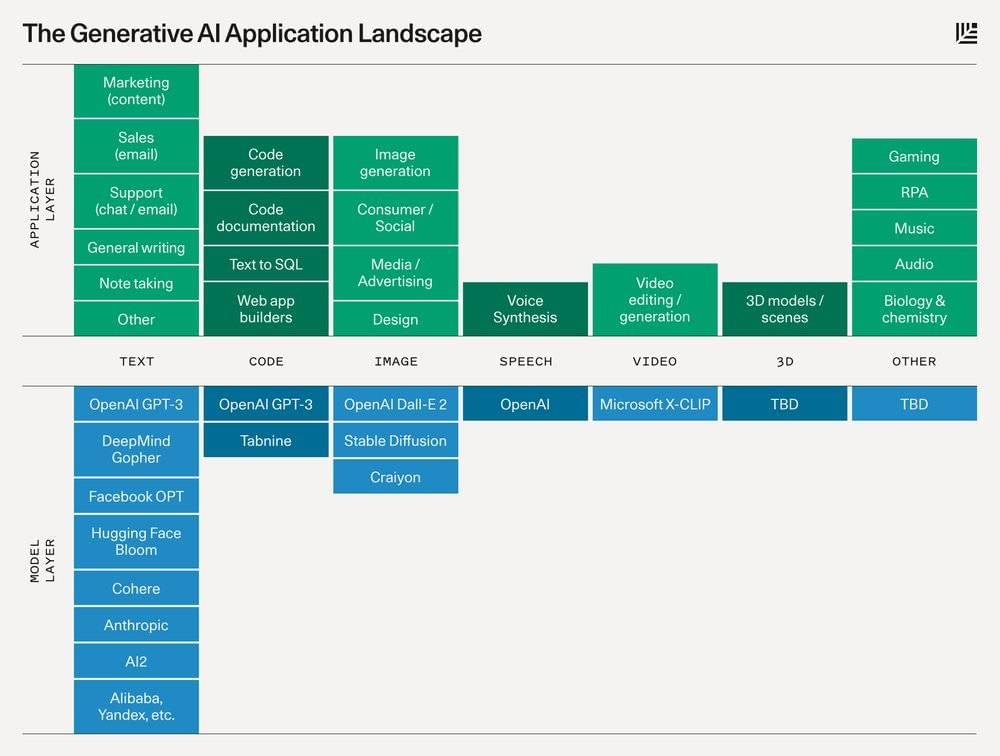
Models
Text is the most advanced domain. However, natural language is hard to get right, and quality matters. Today, the models are decently good at generic short/medium-form writing (but even so, they are typically used for iteration or first drafts). Over time, as the models get better, we should expect to see higher quality outputs, longer-form content, and better vertical-specific tuning.
Code generation is likely to have a big impact on developer productivity in the near term as shown by GitHub CoPilot. It will also make the creative use of code more accessible to non developers.
Images are a more recent phenomenon, but they have gone viral: it’s much more fun to share generated images on Twitter than text! We are seeing the advent of image models with different aesthetic styles, and different techniques for editing and modifying generated images.
Speech synthesis has been around for a while (hello Siri!) but consumer and enterprise applications are just getting good. For high-end applications like film and podcasts the bar is quite high for one-shot human quality speech that doesn’t sound mechanical. But just like with images, today’s models provide a starting point for further refinement or final output for utilitarian applications.
Video and 3D models are further behind. People are excited about these models’ potential to unlock large creative markets like cinema, gaming, VR, architecture and physical product design. We should expect to see foundational 3D and video models in the next 1-2 years.
Other domains: There is fundamental model R&D happening in many fields, from audio and music to biology and chemistry (generative proteins and molecules, anyone?).
The below chart illustrates a timeline for how we might expect to see fundamental models progress and the associated applications that become possible. 2025 and beyond is just a guess.
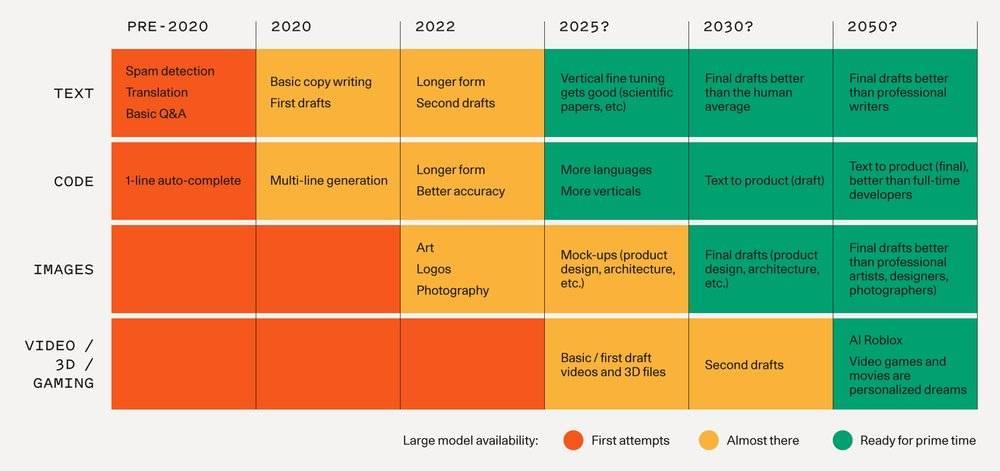
Applications
Here are some of the applications we are excited about. There are far more than we have captured on this page, and we are enthralled by the creative applications that founders and developers are dreaming up.
Copywriting: The growing need for personalized web and email content to fuel sales and marketing strategies as well as customer support are perfect applications for language models. The short form and stylized nature of the verbiage combined with the time and cost pressures on these teams should drive demand for automated and augmented solutions.
Vertical specific writing assistants: Most writing assistants today are horizontal; we believe there is an opportunity to build much better generative applications for specific end markets, from legal contract writing to screenwriting. Product differentiation here is in the fine-tuning of the models and UX patterns for particular workflows.
Code generation: Current applications turbocharge developers and make them much more productive: GitHub Copilot is now generating nearly 40% of code in the projects where it is installed. But the even bigger opportunity may be opening up access to coding for consumers. Learning to prompt may become the ultimate high-level programming language.
Art generation: The entire world of art history and pop cultures is now encoded in these large models, allowing anyone to explore themes and styles at will that previously would have taken a lifetime to master.
Gaming: The dream is using natural language to create complex scenes or models that are riggable; that end state is probably a long way off, but there are more immediate options that are more actionable in the near term such as generating textures and skybox art.
Media/Advertising: Imagine the potential to automate agency work and optimize ad copy and creative on the fly for consumers. Great opportunities here for multi-modal generation that pairs sell messages with complementary visuals.
Design: Prototyping digital and physical products is a labor-intensive and iterative process. High-fidelity renderings from rough sketches and prompts are already a reality. As 3-D models become available the generative design process will extend up through manufacturing and production—text to object. Your next iPhone app or sneakers may be designed by a machine.
Social media and digital communities: Are there new ways of expressing ourselves using generative tools? New applications like Midjourney are creating new social experiences as consumers learn to create in public.
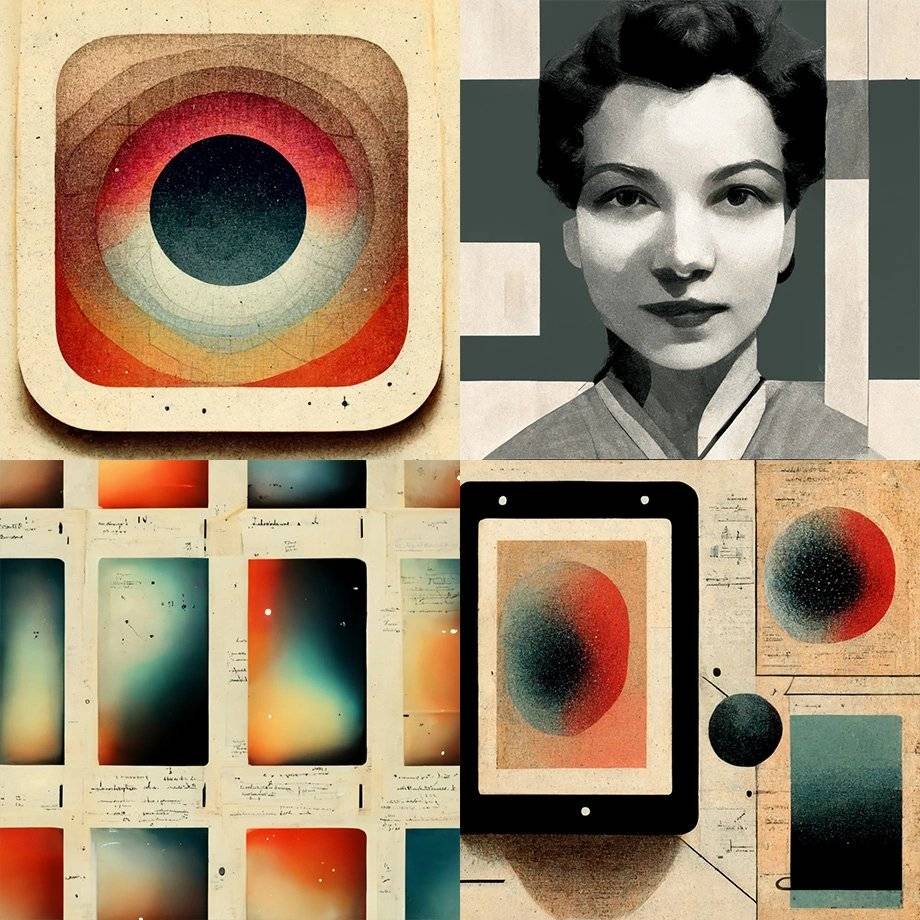
Anatomy of a Generative AI Application
What will a generative AI application look like? Here are some predictions.
Intelligence and model fine-tuning
Generative AI apps are built on top of large models like GPT-3 or Stable Diffusion. As these applications get more user data, they can fine-tune their models to: 1) improve model quality/performance for their specific problem space and; 2) decrease model size/costs.
We can think of Generative AI apps as a UI layer and “little brain” that sits on top of the “big brain” that is the large general-purpose models.
Form Factor
Today, Generative AI apps largely exist as plugins in existing software ecosystems. Code completions happen in your IDE; image generations happen in Figma or Photoshop; even Discord bots are the vessel to inject generative AI into digital/social communities.
There are also a smaller number of standalone Generative AI web apps, such as Jasper and Copy.ai for copywriting, Runway for video editing, and Mem for note taking.
A plugin may be an effective wedge into bootstrapping your own application, and it may be a savvy way to surmount the chicken-and-egg problem of user data and model quality (you need distribution to get enough usage to improve your models; you need good models to attract users). We have seen this distribution strategy pay off in other market categories, like consumer/social.
Paradigm of Interaction
Today, most Generative AI demos are “one-and-done”: you offer an input, the machine spits out an output, and you can keep it or throw it away and try again. Increasingly, the models are becoming more iterative, where you can work with the outputs to modify, finesse, uplevel and generate variations.
Today, Generative AI outputs are being used as prototypes or first drafts. Applications are great at spitting out multiple different ideas to get the creative process going (e.g. different options for a logo or architectural design), and they are great at suggesting first drafts that need to be finessed by a user to reach the final state (e.g. blog posts or code autocompletions). As the models get smarter, partially off the back of user data, we should expect these drafts to get better and better and better, until they are good enough to use as the final product.
Sustained Category Leadership
The best Generative AI companies can generate a sustainable competitive advantage by executing relentlessly on the flywheel between user engagement/data and model performance.
To win, teams have to get this flywheel going by 1) having exceptional user engagement → 2) turning more user engagement into better model performance (prompt improvements, model fine-tuning, user choices as labeled training data) → 3) using great model performance to drive more user growth and engagement.
They will likely go into specific problem spaces (e.g., code, design, gaming) rather than trying to be everything to everyone. They will likely first integrate deeply into applications for leverage and distribution and later attempt to replace the incumbent applications with AI-native workflows. It will take time to build these applications the right way to accumulate users and data, but we believe the best ones will be durable and have a chance to become massive.
Hurdles and Risks
Despite Generative AI’s potential, there are plenty of kinks around business models and technology to iron out. Questions over important issues like copyright, trust & safety and costs are far from resolved.
Eyes Wide Open
Generative AI is still very early. The platform layer is just getting good, and the application space has barely gotten going.
To be clear, we don’t need large language models to write a Tolstoy novel to make good use of Generative AI. These models are good enough today to write first drafts of blog posts and generate prototypes of logos and product interfaces. There is a wealth of value creation that will happen in the near-to-medium-term.
This first wave of Generative AI applications resembles the mobile application landscape when the iPhone first came out—somewhat gimmicky and thin, with unclear competitive differentiation and business models. However, some of these applications provide an interesting glimpse into what the future may hold. Once you see a machine produce complex functioning code or brilliant images, it’s hard to imagine a future where machines don’t play a fundamental role in how we work and create.
If we allow ourselves to dream multiple decades out, then it’s easy to imagine a future where Generative AI is deeply embedded in how we work, create and play: memos that write themselves; 3D print anything you can imagine; go from text to Pixar film; Roblox-like gaming experiences that generate rich worlds as quickly as we can dream them up.
While these experiences may seem like science fiction today, the rate of progress is incredibly high—we have gone from narrow language models to code auto-complete in several years—and if we continue along this rate of change and follow a “Large Model Moore’s Law,” then these far-fetched scenarios may just enter the realm of the possible.
PS: This piece was co-written with GPT-3. GPT-3 did not spit out the entire article, but it was responsible for combating writer’s block, generating entire sentences and paragraphs of text, and brainstorming different use cases for generative AI. Writing this piece with GPT-3 was a nice taste of the human-computer co-creation interactions that may form the new normal. We also generated illustrations for this post with Midjourney, which was SO MUCH FUN!
以下为中文版:
人类擅长分析事物,而机器在这方面甚至做得就更好了。机器可以分析一组数据,并在其中找到许多用例(use case)的模式,无论是欺诈还是垃圾邮件检测,预测你的发货时间或预测该给你看哪个TikTok视频,它们在这些任务中变得越来越聪明。这被称为“分析型AI(Analytical AI)”,或传统AI。
但是人类不仅擅长分析事物,我们也擅长创造。我们写诗,设计产品,制作游戏,编写代码。直到最近,机器还没有机会在创造性工作上与人类竞争——它们被降格为只做分析和机械性的认知工作。但最近,机器开始尝试创造有意义和美丽的东西,这个新类别被称为“生成式AI(Generative AI)”,这意味着机器正在生成新的东西,而不是分析已经存在的东西。
生成式AI正在变得不仅更快、更便宜,而且在某些情况下比人类创造的更好。从社交媒体到游戏,从广告到建筑,从编程到平面设计,从产品设计到法律,从市场营销到销售,每一个原来需要人类创作的行业都等待着被机器重新创造。某些功能可能完全被生成式AI取代,而其他功能则更有可能在人与机器之间紧密迭代的创作周期中蓬勃发展。但生成式AI应该在广泛的终端市场上解锁更好、更快、更便宜的创作。人们期待的梦想是:生成式AI将创造和知识工作的边际成本降至零,产生巨大的劳动生产率和经济价值,以及相应的市值。
生成式AI可以处理的领域包括了知识工作和创造性工作,而这涉及到数十亿的人工劳动力。生成式AI可以使这些人工的效率和创造力至少提高10%,它们不仅变得更快和更高效,而且比以前更有能力。因此,生成式AI有潜力产生数万亿美元的经济价值。
为什么是现在?
生成式AI与更广泛的AI有着相同的“为什么是现在(Why now)”的原因:更好的模型,更多的数据,更多的算力。这个类别的变化速度比我们所能捕捉到的要快,但我们有必要在大背景下回顾一下最近的历史。
第1波浪潮:小模型(small models)占主导地位(2015年前),小模型在理解语言方面被认为是“最先进的”。这些小模型擅长于分析任务,可以用于从交货时间预测到欺诈分类等工作。但是,对于通用生成任务,它们的表达能力不够。生成人类级别的写作或代码仍然是一个白日梦。
第2波浪潮:规模竞赛(2015年-至今),Google Research的一篇里程碑式的论文(Attention is All You Need https://arxiv.org/abs/1706.03762)描述了一种用于自然语言理解的新的神经网络架构,称为transformer,它可以生成高质量的语言模型,同时具有更强的并行性,需要的训练时间更少。这些模型是简单的学习者,可以相对容易地针对特定领域进行定制。
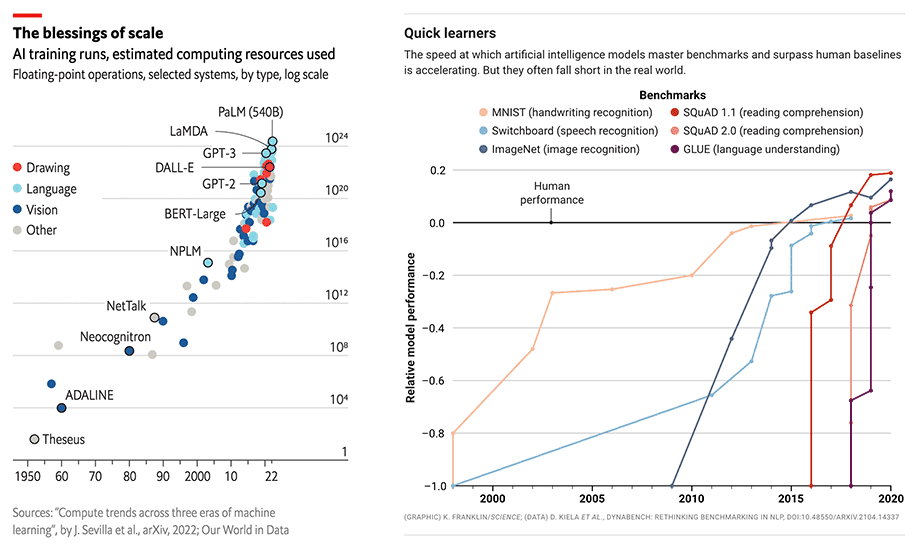
果不其然,随着模型越来越大,它们开始可以输出达到人类水平的结果,然后是超人的结果。从2015年到2020年,用于训练这些模型的计算量增加了6个数量级,其结果在书写、语音、图像识别、阅读和语言理解方面超过了人类的表现水平。OpenAI的GPT-3表现尤其突出:该模型的性能比GPT-2有了巨大的飞跃,并且从代码生成到笑话编写的任务中都提供了出色的Twitter demo来证明。
尽管所有的基础研究都取得了进展,但这些模型并不普遍。它们庞大且难以运行(需要特别的GPU配置),不能被更多人广泛触达使用(不可用或只进行封闭测试),而且作为云服务使用成本昂贵。尽管存在这些限制,最早的生成式AI应用程序也已经开始进入竞争。
第3波浪潮:更好、更快和更便宜(2022+),算力变得更便宜,新技术,如扩散模型(diffusion models),降低了训练和运行所需的成本。研究人员继续开发更好的算法和更大的模型。开发人员的访问权限从封闭测试扩展到开放测试,或者在某些情况下扩展到开源。
对于那些渴望接触LLMs(Large Language Model 大语言模型)的开发人员来说,探索和应用开发的闸门现在已经打开,应用开始大量涌现。
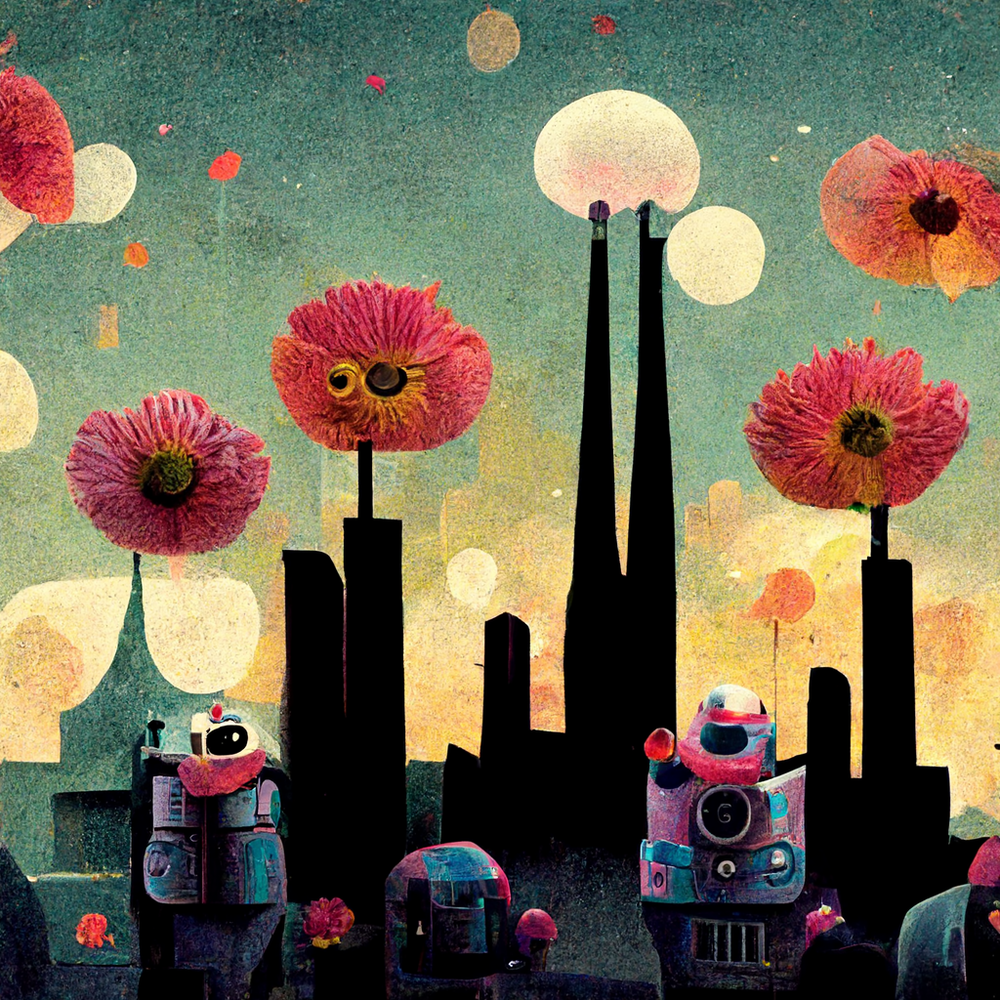
第4波浪潮:杀手级应用出现(现在),随着平台层的稳固,模型继续变得更好、更快和更便宜,模型的获取趋于免费和开源,应用层的创造力已经成熟。
正如移动设备通过GPS、摄像头和网络连接等新功能释放了新类型的应用程序一样,我们预计这些大型模型将激发生成式AI应用程序的新浪潮。就像十年前移动互联网的拐点被一些杀手级应用打开了市场一样,我们预计生成式AI的杀手级应用程序也会出现,比赛开始了。
市场格局
下面是一个示意图,说明了为每个类别提供动力的平台层,以及将在其上构建的潜在应用程序类型。
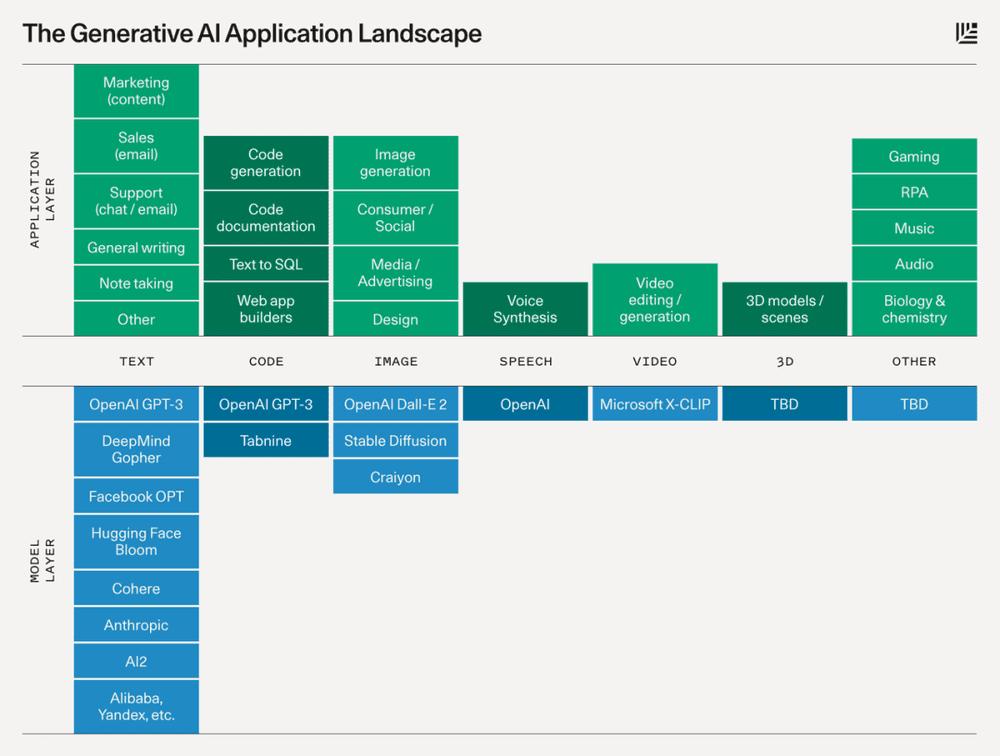
模型
文本(Text)是最先进的领域,然而,自然语言很难被正确使用并且质量很重要。如今,这些模型在一般的中短篇形式的写作中相当出色(但即便如此,它们通常用于迭代或初稿)。随着时间的推移,模型变得越来越好,我们应该期望看到更高质量的输出、更长形式的内容和更好的垂直领域深度。
代码生成(Code generation)可能会在短期内对开发人员的生产力产生很大的影响,正如GitHub CoPilot所表现的那样。此外,代码生成还将使非开发人员更容易创造性地使用代码。
图片(Images)是最近才出现的现象,但它们已经像病毒一样传播开来。在Twitter上分享生成的图片比文本有趣得多!我们正在看到具有不同美学风格的图像模型和用于编辑和修改生成图像的不同技术在陆续出现。
语音合成(Speech synthesis)已经出现一段时间了,但消费者和企业应用才刚刚起步。对于像电影和播客这样的高端应用程序来说,听起来不机械的,具有人类质量的语音是相当高的门槛。但就像图像一样,今天的模型为进一步优化或实现应用的最终输出提供了一个起点。
视频和3D模型则远远落后,人们对这些模型的潜力感到兴奋,因为它们可以打开电影、游戏、虚拟现实、建筑和实物产品设计等大型创意市场。我们应该期待在未来1-2年内看到基础的3D和视频模型的出现。
还有很多其他领域,比如从音频和音乐到生物和化学等等,都在进行基础模型的研发。下面这张图是基本模型进展和相关应用程序成为可能的时间表,其中2025年及以后的部分只是一个猜测。
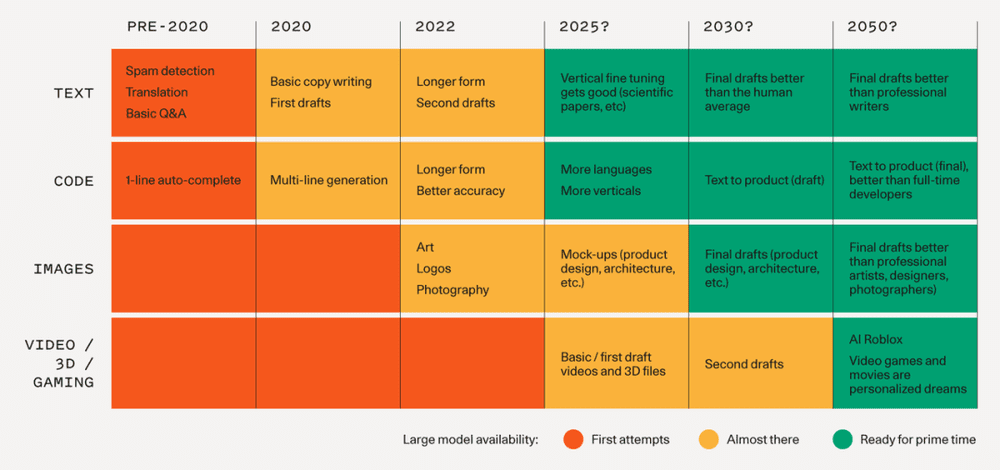
应用程序
以下是一些让我们感到兴奋的应用,这仅仅只是一部分,实际上的应用要比我们所捕捉到的多得多,我们被创始人和开发人员所梦想的创造性应用程序所吸引。
文案(Copywriting):越来越多的人需要个性化的网页和电子邮件内容来推动销售和营销策略以及客户支持,这是语言模型的完美应用。这些文案往往形式简单,并且都有固定的模版,加上这些团队的时间和成本压力,应该会大大推动对自动化和增强解决方案的需求。
垂直行业的写作助手(Vertical specific writing assistants):现在大多数写作助手都是通用型的,我们相信为特定的终端市场构建更好的生成式应用程序有着巨大机会,比如从法律合同编写到剧本编写。这里的产品差异化体现在针对特定工作流的模型和UX交互的微调。
代码生成(Code generation):当前的应用程序推动了开发人员的发展,使他们的工作效率大大提高。在安装了Copilot的项目中,它生成了近40%的代码。但更大的机会可能是为C端消费者赋能编程开发能力,学习提示(learning to prompt)可能会成为最终的高级编程语言。
艺术生成(Art generation):整个艺术史和流行文化的世界现在都被编码进了这些大型模型中,这将允许任何人随意探索在以前可能需要花人一辈子的时间才能掌握的主题和风格。
游戏(Gaming):在这方面的梦想是使用自然语言创建复杂的场景或可操纵的模型,这个最终状态可能还有很长一段路要走,但在短期内有更直接的选择,如生成纹理和天空盒艺术(skybox art)。
媒体/广告(Media/Advertising):想象一下自动化代理工作的潜力,为消费者实时优化广告文案和创意。多模态生成的绝佳机会是将销售信息与互补的视觉效果结合起来。
设计(Design):设计数字和实物产品的原型是一个劳动密集型的迭代过程,AI根据粗略的草图和提示来制作高保真的效果图已经成为现实。随着3D模型的出现,生成设计的过程将从制造和生产延伸到实物,你的下一个iPhone APP或运动鞋可能是由机器设计的。
社交媒体和数字社区(Social media and digital communities):是否存在使用生成工具表达自我的新方式?随着Midjourney等新应用学会了像人类一样在社交网络上创作,这将创造新的社交体验。
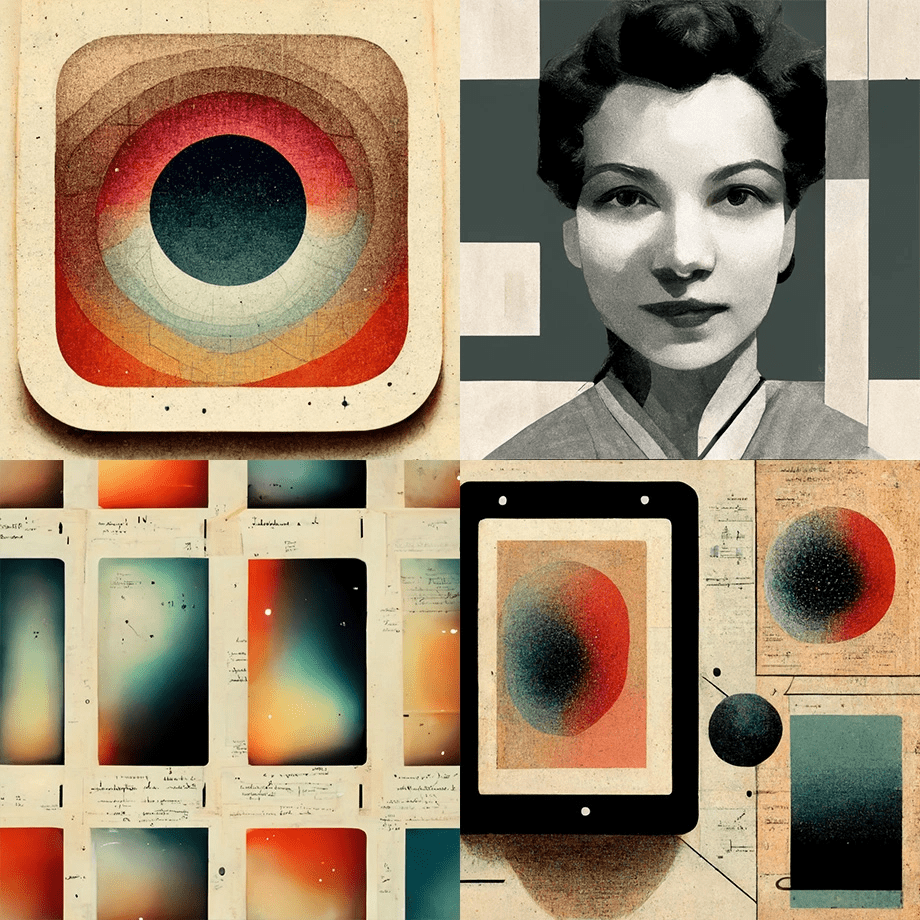
生成式AI应用的解析
生成式AI应用程序会是什么样子?以下是一些预测:
智能和模型微调
生成式AI应用是建立在GPT-3或Stable Diffusion等大型模型之上的,随着这些应用获得更多的用户数据,它们可以对模型进行微调,一方面针对特定的问题空间改进模型质量和性能,另外一方面减少模型的大小和成本。
我们可以把生成式AI应用看作一个UI层和位于大型通用模型“大大脑(big brain)”之上的“小大脑(little brain)”。
形成的因素
如今,生成式AI应用在很大程度上以插件的形式存在于现有的软件生态系统中。比如代码生成在你的IDE中,图像生成在Figma或Photoshop中,甚至Discord机器人也是将生成AI放在数字社交社区里的工具。
还有少量独立的生成式AI Web应用,如在文案方面有Jasper和Copy.ai,在视频剪辑方面有Runway,在做笔记方面有Mem。
插件的形式可能是生成式AI应用在早期比较好的切入点,它可以克服用户数据和模型质量方面面临的“先有鸡还是先有蛋”的问题(这里具体指的是:一方面需要分发来获得足够多的使用数据,从而来改进模型,另外一方面又需要好的模型来吸引用户)。我们已经看到这种策略在其他市场类别中取得了成功,如消费者和社交市场。
交互范式
如今,大多数生成式AI演示都是“一次性”的:你提供一个输入,机器吐出一个输出,你可以保留它或扔掉它,然后再试一次。未来,模型将会支持迭代,你可以使用输出来修改、调整、升级和生成变化。
如今,生成式AI输出被用作原型或初稿。应用程序非常擅长抛出多个不同的想法,以使创作过程继续(比如一个logo或建筑设计的不同选项),它们也非常擅长给出初稿,但需要用户最终润色来定稿(比如博客帖子或代码自动完成)。随着模型变得越来越智能,同时部分借助于用户数据,我们应该期待这些草稿会变得越来越好,直到它们足够好,可以用作最终产品。
持续的行业领导力
最好的生成式AI公司可以通过在用户粘性、数据和模型性能之间形成的飞轮来产生可持续的竞争优势。为了取得胜利,团队必须通过以下方法来实现这个飞轮:
拥有出色的用户粘性→将更多的用户粘性转化为更好的模型性能(及时改进、模型微调、把用户选择作为标记训练数据)→使用出色的模型性能来推动更多的用户增长和留存。
他们可能会专注于特定的领域(如代码、设计和游戏),而不是试图解决所有人的问题。他们可能首先将深度集成到现有的应用程序中,以便在此基础上利用和分发自己的程序,然后尝试用AI原生工作流替换现有的应用程序。用正确的方式构建这些应用来积累用户和数据是需要时间的,但我们相信最好的应用将会是持久的,并有机会变得庞大。
困难和风险
尽管生成式AI具有巨大的潜力,但在商业模式和技术方面仍有许多问题需要解决。比如版权、信任、安全和成本等重要问题还亟待解决。
放开视野
生成式AI仍然非常早期。平台层刚刚有起色,而应用层领域才刚刚起步。
需要明确的是,我们不需要利用大型语言模型的生成式AI来编写托尔斯泰小说。这些模型现在已经足够好了,可以用来写博客文章的初稿,以及生成logo和产品界面的原型,这在中短期内将会创造大量的价值。
生成式AI应用的第一波浪潮,类似于iPhone刚出现时的移动应用场景——有些噱头但比较单薄,竞争差异化和商业模式不明确。然而,其中一些应用程序提供了一个有趣的视角,让我们可以一窥未来可能会发生什么。一旦你看到了机器可以产生复杂的功能代码或精彩的图片,你就很难想象未来机器在我们的工作和创造中不再发挥作用。
如果我们允许自己梦想几十年后,那么很容易想象一个未来,生成式AI将深深融入我们的工作、创作和娱乐方式:备忘录可以自己写,3D打印任何你能想象的东西,从文字到皮克斯电影,像Roblox类似的游戏体验来快速创造出丰富的世界。
虽然这些在今天看起来像是科幻小说,但科技进步的速度是惊人的。从微小(narrow)的语言模型到代码自动生成只用了几年时间,如果我们继续沿着这个变化的速度,并遵循“大模型摩尔定律(Large Model Moore's Law)”,那么这些遥不可及的场景就会变得触手可及。
本文来自:红杉,编译:深思圈